
Tomato Health and Fruit Count Check Using Smart Algorithm
Jim Crocker
18th April, 2024
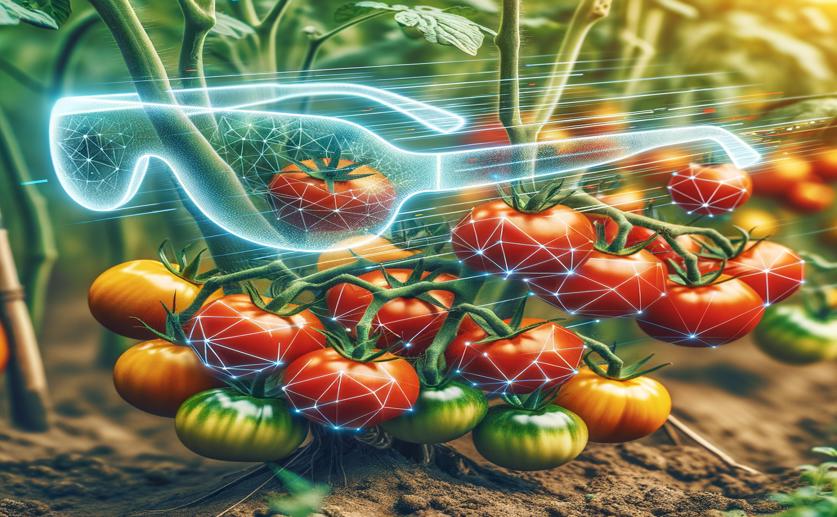
Image Source: Natural Science News, 2024
Key Findings
- In Jiangsu, a new system uses AI to detect tomato diseases and count fruits
- The system's YOLO-TGI model identifies leaf diseases with high accuracy and low resource use
- For counting tomatoes, YOLO-TGI-S paired with Byte-Track is fast and precise
AgricultureBiotechPlant Science
References
Main Study
1) Toward Real Scenery: A Lightweight Tomato Growth Inspection Algorithm for Leaf Disease Detection and Fruit Counting.
Published 17th April, 2024
https://doi.org/10.34133/plantphenomics.0174
Related Studies
2) Tomato Diseases and Pests Detection Based on Improved Yolo V3 Convolutional Neural Network.
3) Deep learning.
4) A Precise Image-Based Tomato Leaf Disease Detection Approach Using PLPNet.
5) Tomato Fruit Detection and Counting in Greenhouses Using Deep Learning.