
LeTra: Tracking Plant Growth with AI and Smart Overlap Measures
Jim Crocker
18th January, 2024
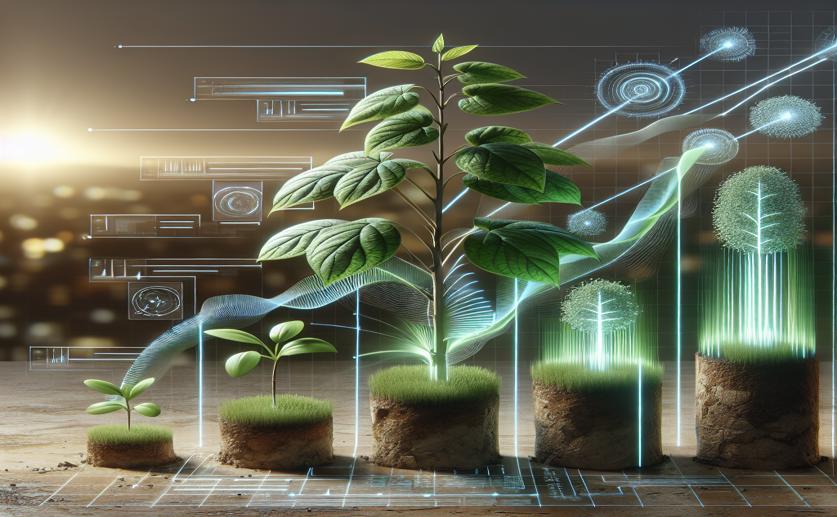
Image Source: Natural Science News, 2024
References
Main Study
1) LeTra: a leaf tracking workflow based on convolutional neural networks and intersection over union.
Published 17th January, 2024
https://doi.org/10.1186/s13007-024-01138-x