
Improving Winter Wheat Forecasts Using Genetics, Traits, and Environmental Data
Jim Crocker
1st June, 2024
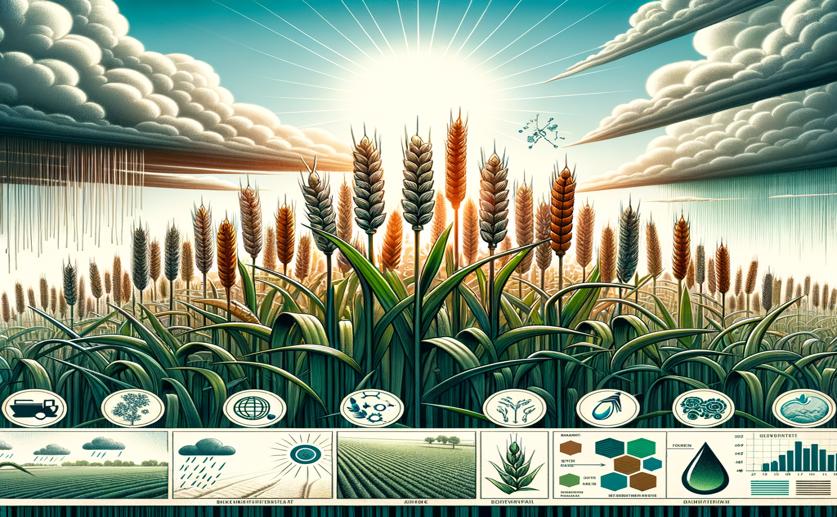
Image Source: Natural Science News, 2024
Key Findings
- The study from Universidad de Colima focused on improving genomic selection (GS) models for soft white winter wheat by integrating environmental information
- Incorporating environmental data into GS models significantly improved prediction accuracy, with an average gain of 49.19% in normalized root mean square error (NRMSE)
- The improvement in prediction accuracy varied across data sets, ranging from 5.68% to 60.36%, demonstrating the substantial effect of including environmental information
AgricultureEnvironmentGenetics
References
Main Study
1) Enhancing winter wheat prediction with genomics, phenomics and environmental data
Published 31st May, 2024
https://doi.org/10.1186/s12864-024-10438-4
Related Studies
2) Genome and Environment Based Prediction Models and Methods of Complex Traits Incorporating Genotype × Environment Interaction.
3) Factor analytic mixed models for the provision of grower information from national crop variety testing programs.
4) Genomic Selection in Plant Breeding: Methods, Models, and Perspectives.