
Forecasting Tomato Quality with Genetic Testing
Jim Crocker
28th March, 2024
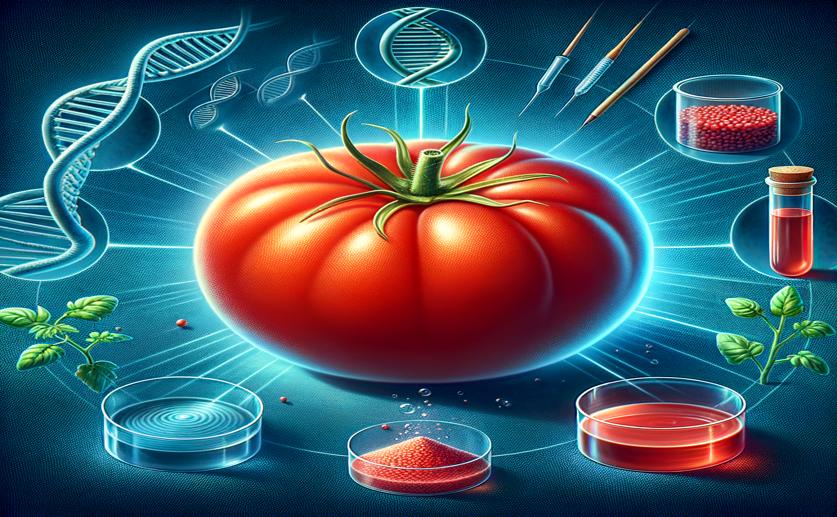
Image Source: Natural Science News, 2024
Key Findings
- Study at Sejong University improved tomato breeding by predicting traits like size and sweetness
- Random forest model was best for predicting tomato weight, width, and skin thickness
- Fewer genetic markers (SNPs) can predict traits more accurately, making breeding more cost-effective
GeneticsPlant ScienceAgriculture
References
Main Study
1) Prediction accuracy of genomic estimated breeding values for fruit traits in cultivated tomato (Solanum lycopersicum L.).
Published 27th March, 2024
https://doi.org/10.1186/s12870-024-04934-8
Related Studies
2) Genome-wide association study identifies QTL for eight fruit traits in cultivated tomato (Solanum lycopersicum L.).
3) Genome-wide association mapping in tomato (Solanum lycopersicum) is possible using genome admixture of Solanum lycopersicum var. cerasiforme.
4) Mapping of two suppressors of OVATE (sov) loci in tomato.