
Predicting Corn Water Use with Advanced AI Using Weather and Crop Data
Jim Crocker
4th March, 2024
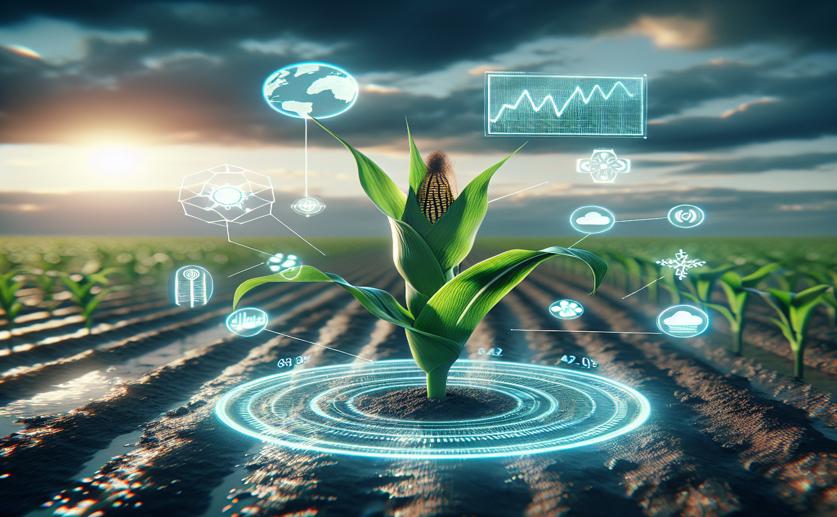
Image Source: Natural Science News, 2024
Key Findings
- In Northern China, a new model predicts maize water loss to the air with improved accuracy
- The model combines weather, soil, and crop data, optimized by AI algorithms
- The best AI-enhanced model was 2.7-4.8% more accurate than previous methods
AgricultureBiotechPlant Science
References
Main Study
1) Estimating maize evapotranspiration based on hybrid back-propagation neural network models and meteorological, soil, and crop data.
Published 10th January, 2024
https://doi.org/10.1007/s00484-023-02608-y
Related Studies
2) Modelling the reference crop evapotranspiration in the Beas-Sutlej basin (India): an artificial neural network approach based on different combinations of meteorological data.
3) Artificial intelligence models versus empirical equations for modeling monthly reference evapotranspiration.